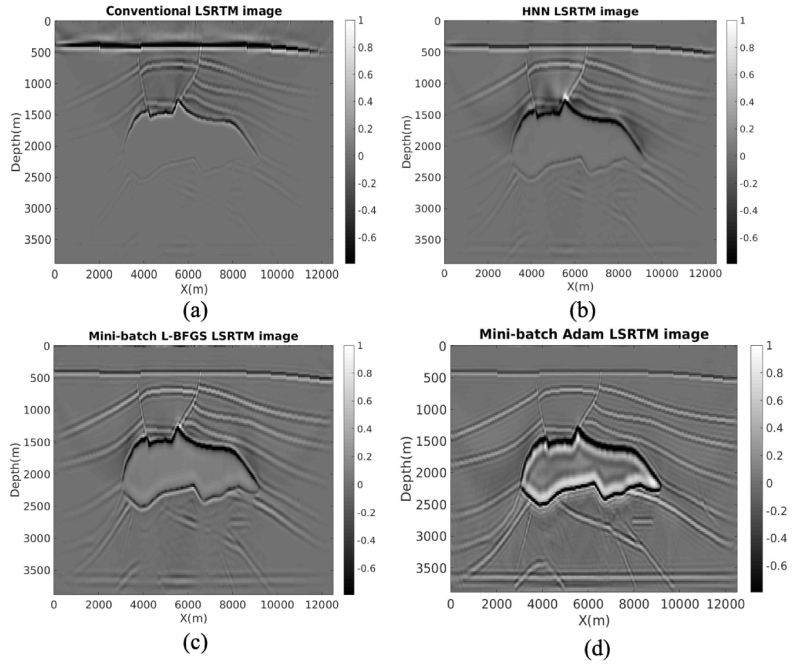
The final UTIG seminar of the Fall semester is reserved for graduate students to practice their presentations for AGU Fall. This year’s practice talks will be given by UTIG grad students Xian Wu, Kelly Olsen and Janaki Vamaraju. We asked each student to give us a sneak-preview into what they’ll be talking about and why they’re excited about sharing their research with the science community.
The seminar takes place at 10:30am (CST) on Friday, December 6 at UTIG. Click here to watch the seminar online.
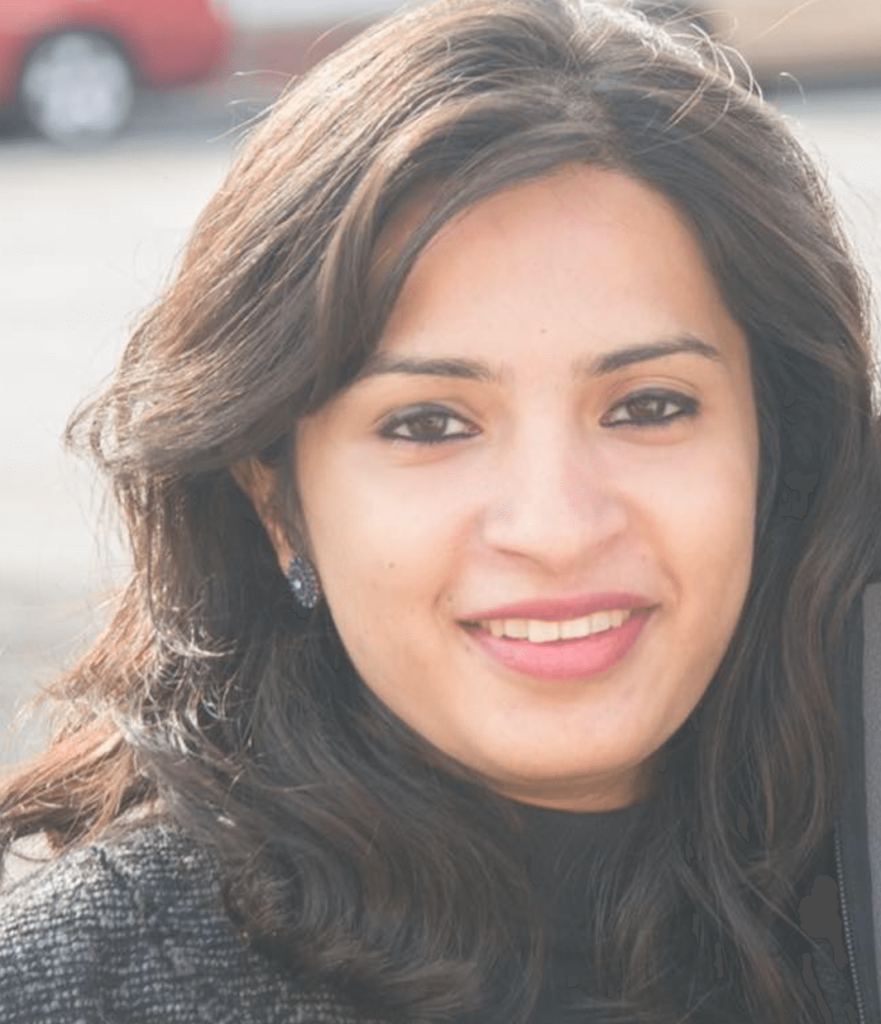
Name: Janaki Vamaraju
Degree: Computational Geophysics PhD
Funding: Ewing/Worzel UTIG Fellowship, NSF, EDGER Forum
Expected Graduation: Fall 2019
Hi Janaki. Congratulations on defending your PhD! No doubt you’re planning something brilliant for the UTIG Seminar. What will you be talking about?
I’ll be talking about using machine learning tools to improve the efficiency of inverse problems in seismic imaging algorithms.
You do what to who exactly?
Seismic modeling and imaging are sophisticated tools that are applied to complicated subsurface geological settings. However, these problems are, in general, difficult to solve accurately.
Good thing we have a supercomputer next door!
Several actually. Numerical simulations in exploration geophysics use high-performance computing capabilities, like those offered by TACC, to analyze data and observations. My work proposes efficient techniques to overcome some of these challenges.
But this is Texas. Surely even the supercomputers are bigger?
Even with advanced supercomputing facilities, the computational cost and complexity of calculations in geophysics tend to increase rapidly with the size of the system you are investigating, whether that means a larger areas or higher resolution. By developing cost effective algorithms for simulations and modeling we can do more calculations, which means we can help accelerate geoscientific discoveries. Also time is money. So, this line of research is very important for reducing computation costs and improving operational efficiency.
That’s very sound reasoning. But what do you do with it?
The work I do focuses on real world applications. For example, you might use the methods I discuss to find fractures in the Earth’s subsurface, which are important because they greatly influence the porosity and permeability of an oil or gas reservoir. Detecting fractures from seismic data is a challenging problem, however, because seismic resolution is low and the geology around reservoirs can be very complex. The challenge is to take what we know about the physical properties of fractures and develop novel numerical models that can accurately model how seismic waves pass through fractures and are also computationally very efficient. These methods are critically important in hydrocarbon exploration, especially for drilling, well completion and reservoir management, but also for unconventional reservoirs.
Are there other uses besides hydrocarbon exploration?
All these techniques fall under the theory of computational science and applied mathematics and are very transferable. For example, you can use it in global seismology to study volcanoes, earthquakes and ground water. The method can also be used to develop geothermal reservoirs and in carbon storage techniques like CO2 sequestration. You can also use the same numerical methods in biomedical imaging by using non-invasive sensors to look for tumors. It’s the same idea, just a very different scale and physics.
So how good is your approach?
My work focuses on two areas; introducing hybrid numerical methods that can simulate seismic wave propagation in fractured media, and developing cost-effective solutions for seismic imaging. The hybrid finite element techniques I developed gave a 25%-30% reduction in computation time and proved to be especially suitable for large scale problems, such as 3D simulations of the subsurface where you might find intersecting fractures and hydraulic fractures. The machine learning methods that I developed for seismic imaging is at least 10 times more computationally efficient than traditional methods, and works well with noisy and sparse data, which is often the case with exploration geophysics!
How do you feel about giving a UTIG seminar talk?
It’s very important to share and receive feedback on your work. The UTIG seminar is a great avenue for exchange of ideas and knowledge and I’m really pleased to be giving a talk.
Now that you’re ready to graduate what’s next?
I want to continue developing machine learning methods to solve physics based problems.
Janaki has published two papers, in the Journal of Computational Physics, and the journal Interpretation, and has three more in the pipeline. For now, she wants to continue solving problems in computational science and will soon begin work in the energy industry.
Janaki’s UTIG Seminar talk, which she will also present at AGU Fall 2019, is titled “Mini-batch Least-Squares Reverse Time Migration In A Deep Learning Framework”.
Good luck with your talks and good luck in the future Janaki!