By Freja Cini & Constantino Panagopulos
GEOPHYSICS Q&A
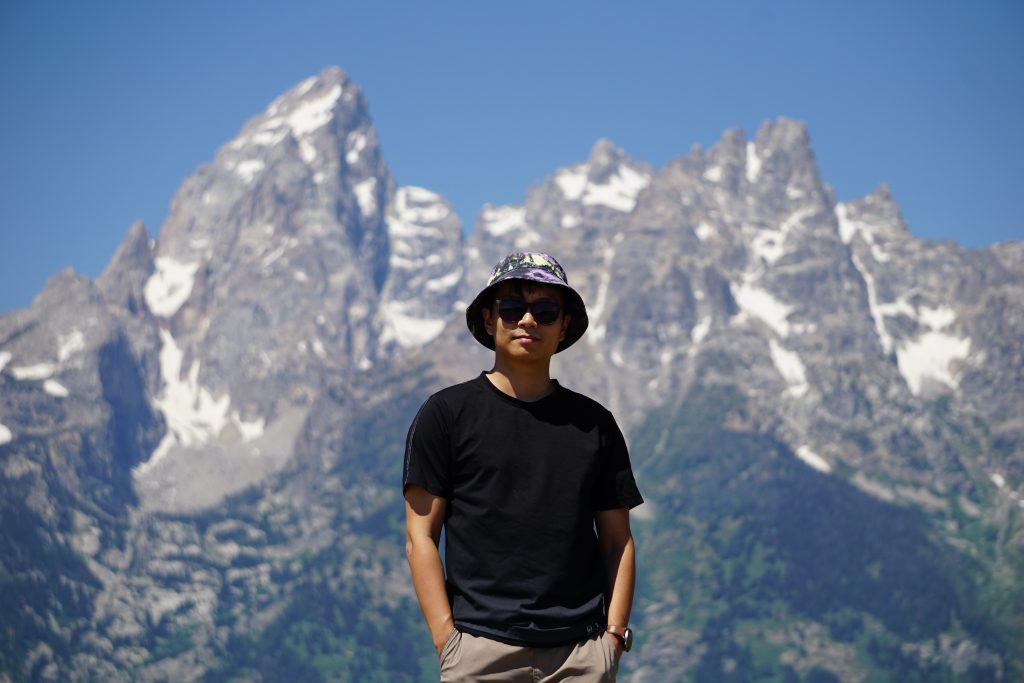
Enze Zhang is the author of AutoTerm, a computer program that uses artificial intelligence to recognize the ocean-facing edges of glaciers — known as the terminus — in satellite images. Zhang’s terminus-recognition technology is providing advance reconnaissance of Greenland’s glaciers for an upcoming research expedition led by The University of Texas at Austin. The high-stakes-mission will send a submersible robot to the glaciers’ underwater ice walls and retrieve hard-to-reach data about climate change and future sea level rise.
Zhang developed AutoTerm during his time as distinguished postdoctoral fellow of the University of Texas Institute for Geophysics. AutoTerm’s code and the accompanying database of glacial termini are publicly available and have become valuable resources in the race to understand the stability of Greenland’s ice sheet.
We caught up with Zhang at the end of his UTIG postdoc as he was preparing to depart Austin and continue his research at the Hong Kong University of Science and Technology.
Q: Tell me about the glaciers you study and why they’re important.
A: My research is about glaciers in Greenland. When a glacier is retreating, it loses mass and causes the sea level to rise.
The place where the glacier meets the ocean is called the glacier terminus. The terminus is important because it’s where the glacier loses most of its ice and it’s where the glacier directly interacts with the ocean.
If we have a better understanding of variation at the glacier’s terminus, such as how ice calves from the glacier and how it’s dropping into the ocean, we can make better estimates on how much the glacier is contributing to sea level rise.
Q: How do you measure variation in the glacier’s terminus?
A: To investigate variation of the glacier terminus, we need a lot of terminus data. Previously, scientists were manually picking the glacier terminus position from thousands of remote sensing [satellite or aerial] images, which is time consuming and labor intensive. They were sitting in front of the computer, looking at each individual image and clicking the mouse to mark the terminus position.
During my PhD, I had developed a deep learning-based method to automate [the process]. I applied the method [at UTIG] and generated a very comprehensive terminus dataset for Greenland.
With this data, we found some interesting results. For instance, the glaciers’ seasonal changes were not controlled by a single process or a single mechanism. Instead, it is controlled by the interplay of multiple processes.
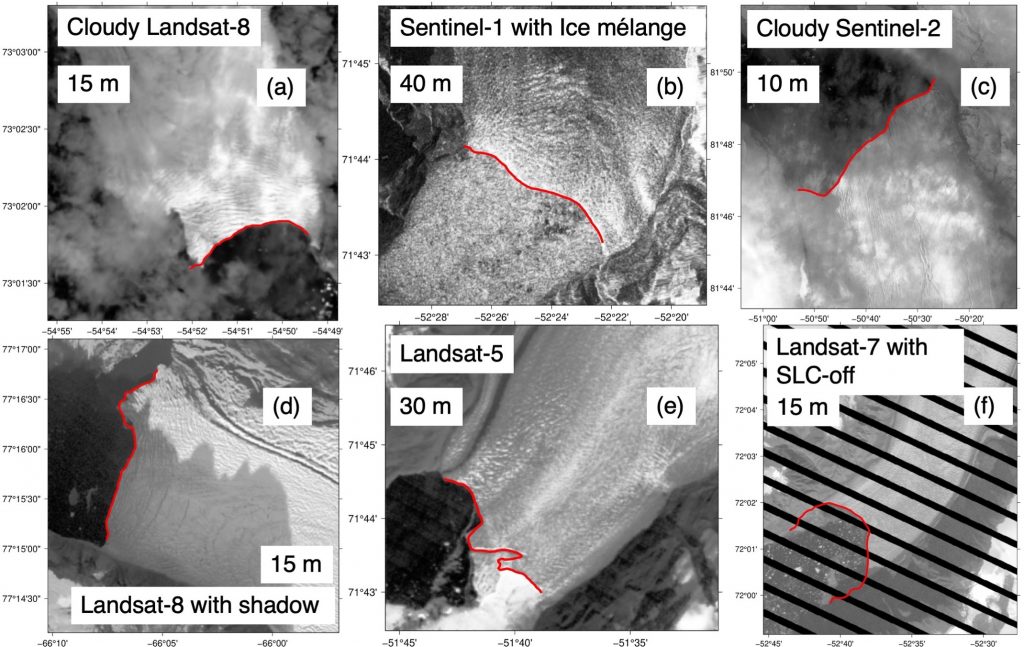
Q: Your deep learning method seems to have really helped with this problem. What kind of issues did you encounter?
A: Automating a large amount of glacier termini using AI requires a high level of generalization, which is the most challenging problem. We solved that by leveraging manually digitized terminus data (images where the glacier’s edge had been marked by hand) to enrich the training dataset and by normalizing the entire dataset via various approaches. Beyond automating terminus extraction itself, there were many technical issues to solve. One was collecting thousands of images of the glaciers in Greenland. [That meant] automating the whole process of collecting photos and editing them to retain the best data quality. We especially focused on the pre-processing and post-processing of the pictures taken of the glaciers.
Deep learning is a powerful tool, but it cannot be perfect all the time. We cannot go through the results it generates one by one—we have to build an automated screening module to detect wrong results and discard them to make sure the final product is clean and friendly to users.
Q: How did you get into this area of research in the first place? What attracted you most to this project?
A: I started working on glaciers when I was an undergraduate, learning how glaciers in Greenland move and how they lose mass into the ocean.
When I went to graduate school, I continued my research on glaciers and found that we rely on a time-consuming and labor-intensive method to study glacial terminus variation. So, I decided to try to find a way to automate this process and have been doing that until now.
Q: Can you tell me a little bit more about your experience as a distinguished postdoc?
A: It was really good. I think the position provided me opportunities to do projects that I’ve always wanted to do. There was also plenty of travel funding provided for postdocs, which we can use to travel to conferences and share our work with colleagues. Through these conferences, we can find funding or make connections with other scientists beyond our institution.
Working with Ginny [Catania] has been really enjoyable because she gave me all the support I needed. Whether it was in terms of doing research, finding job opportunities, or even funding applications, she has been very helpful.
I am now starting my next postdoc at The Hong Kong University of Science. I am generally interested in expanding the application of deep learning in Earth science. My current project aims to improve ocean surface wind data using satellite measurements. The available datasets for ocean surface wind are either low resolution or sparse in their coverage. We’re using AI to kind of fuse the datasets together and give us a high-resolution, global picture of ocean surface wind data that will be especially useful for forecasting typhoons.